Hong Kong Med J 2014;20:194–204 | Number 3, June 2014 | Epub 14 Feb 2014
DOI: 10.12809/hkmj133973
© Hong Kong Academy of Medicine. CC BY-NC-ND 4.0
ORIGINAL ARTICLE
Characteristics of patients readmitted to
intensive care unit: a nested case-control study
OY Tam, FHKCP, FHKAM (Medicine); SM Lam,
FHKCP, FHKAM (Medicine); HP Shum, FHKCP, FHKAM (Medicine); CW Lau,
FHKCP, FHKAM (Medicine); Kenny KC Chan, FHKAM (Anaesthesiology),
FHKCA (Intensive Care); WW Yan, FHKCP, FHKAM (Medicine)
Department of Intensive Care, Pamela Youde
Nethersole Eastern Hospital, Chai Wan, Hong Kong
Corresponding author: Dr OY Tam (toy309@ha.org.hk)
Abstract
Objectives: To evaluate
the pattern of unplanned readmissions to the intensive care unit
and identify patients at risk of readmission.
Design: Nested
case-referent study.
Setting: Tertiary
hospital, Hong Kong.
Patients: A total of 146
patients with unplanned intensive care unit readmission were
compared with 292 control patients who were discharged from the
intensive care unit alive and never readmitted. Cases and
controls were matched for age, gender, and disease severity.
Main outcome measures: Patient
demographics, initial and pre-discharge clinical parameters,
reasons for readmission, and outcomes were studied.
Results: During the
30-month study period, the readmission rate was 5.1%. Readmitted
patients had significantly higher mortality and longer mean
hospital lengths of stay (both P<0.001). Most patients in
this cohort (36.3%) were readmitted for a respiratory cause.
Based on classification tree analysis, postoperative patients
with sepsis (adjusted P=0.043), non-operative septic patients
with fluid gain 24 hours pre-discharge (adjusted P=0.013), and
non-septic patients with increased sputum quantity on discharge
(adjusted P=0.006) were significantly associated with intensive
care unit readmission.
Conclusion: Incomplete
resolution of respiratory conditions remained an important
reason for potentially preventable intensive care unit
readmission. Attention to fluid balance and sputum quantity
before intensive care unit discharge might prevent unplanned
intensive care unit readmission.
New knowledge added by this
study
- The characteristics of patients readmitted to the intensive care unit (ICU) for worsening of pre-existing conditions were different from those readmitted for new complications.
- Risk factors for readmission identified in this study included sepsis during the index admission; positive fluid balance, excessive sputum quantity, weak limb power, higher base excess, and lower haematocrit pre-discharge.
- Early identification of patients at risk and appropriate preventive measures could improve ICU readmission rates and patient outcomes.
Introduction
According to various studies, patient
readmission rates to the intensive care unit (ICU) range from 5% to
10%.1 2 3 4 5
Consistently, readmitted patients had much poorer outcomes, higher
hospital mortality, and their length of stay (LOS) in hospital was
longer.1 3 5 6 7 8 9
Readmissions due to premature ICU discharge are potentially
preventable, and may be attributed to deterioration of the primary
or existing medical condition. Nevertheless, some readmissions are
unavoidable, as there can be occurrence of new complications at
any time after initial ICU discharge. Other factors possibly
contributing to ICU readmissions are organisational factors, such
as ICU occupancy, and availability within a step-down unit.5 10 11Although the early
readmission rate has been advocated as an indicator of ICU
performance, there is little evidence of a correlation between
early ICU readmissions and overall quality of ICU care.2 5 12 13
Risk factors have been identified for ICU readmission.5 7 11 14 15 16 Readmitted patients tend to be older, and
have higher severity scores on initial admission and on discharge.1 5
8 15 17
Recently, Gajic et al18
produced a prediction model with acceptable validity.
This present study aimed to identify
factors associated with unplanned ICU readmissions by comparing
severity-matched cases and controls, whilst focusing on patient
variables at the time of ICU discharge. As it had been repeatedly
shown that the initial disease severity of a patient was
associated with readmissions, we hypothesised that by comparing
severity-matched patients, we might identify modifiable risk
factors for ICU readmissions, especially those that were
potentially preventable.
Methods
The study was carried out in the ICU of
Pamela Youde Nethersole Eastern Hospital, Hong Kong. This was a
20-bed closed system, mixed medical-surgical adult unit, which
provided comprehensive intensive care service to patients in all
specialties, except burns, transplant, and cardiothoracic surgery.
A nested case-control design was therefore used to facilitate data
collection.
Patient selection and data collection
Patients with unplanned ICU readmission
during the same hospitalisation episode were taken as the study
cases. Only the first readmission was used for analysis, whilst
patients who died during their index ICU admission and those with
elective readmissions were excluded. Each study case was compared
with two control patients. Closest matches were selected according
to the order of age (range, ± 5 years), initial disease severity
according to the Acute Physiology and Chronic Health Evaluation
(APACHE) IV risk of death (ROD) [range, ± 5 years], and gender.
When there were more than two matched patients, the two having the
closest date of ICU admission to the case were selected as
controls.
Direct discharge from ICU to home or to
another hospital and patients with documented “Do not resuscitate”
instruction upon ICU discharge were excluded. Data from 1 January
2008 to 31 June 2010 were obtained for all cases and controls
retrospectively, and included their demographic data, functional
status and co-morbidities, pre-discharge physiological parameters
and laboratory findings, treatments and interventions during the
index admission, and time to readmission. The immediate cause of
readmission was determined from detailed review of the medical
record and was categorised to be of new complication (acquired
after ICU discharge) or worsening of a pre-existing condition.
Reasons for readmission were classified into eight major
categories according to the organ system involved.
Definitions
The index ICU admissions were defined as
the first admission of a case, and the only admission of a
control. A patient's pre-existing conditions included the chief
medical problem leading to the index ICU admission and its
complications. Self-care ability was according to the Karnofsky
performance status score.19
Diagnosis of sepsis was based on the clinical judgement of
attending physicians with or without microbiological proof.
Discharges between 09:00 and 17:59 were daytime discharge. The
proportion of ICU beds occupied at time 23:59 of each calendar day
was regarded as the ICU occupancy for that day. Early readmissions
were defined as readmissions within 72 hours of the index admission discharge,
unless stated otherwise.
Statistical analyses
Values were expressed as mean ± standard
deviation (SD) or the number of cases and proportions, as
appropriate. Categorical variables were compared using the Pearson
Chi squared test or Fisher's exact test, as appropriate. The
Student t test or Mann-Whitney U test was used to
compare quantitative data. Binary logistic regression with forward
stepwise elimination was used for multivariate analysis. Predictor
variables of readmission with P≤0.1 in the univariate analysis
were included in the multivariate logistic regression. Variables
with substantial missing data (>15%) were excluded.
At post-hoc analysis, the classification
tree model was employed to identify risks for readmission. This is
a standard data mining statistical tool, using non-parametric
testing to classify cases into subgroups of the dependent
variable, based on the values of the independent variables.
Exhaustive Chi squared Automatic Interaction Detector (CHAID) was
the splitting method. The analysis was conducted in a stepwise
fashion using the Pearson Chi squared test. The predictor variable
with the smallest Bonferroni adjusted P value and yielding the
most significant split was chosen, and nodes were created that
maximised group differences on the outcome. A terminal node was
produced when the smallest adjusted P value for any predictor was
not significant or the number of cases in the child node was
<50. Statistical analyses were conducted using the Statistical
Package for the Social Sciences (Windows version 16.0; SPSS Inc,
Chicago [IL], US).
Results
Patient characteristics are summarised in Tables 1 and 2. There were no statistical significant
differences between readmissions and controls in terms of age,
APACHE IV score, APACHE IV acute physiology score, and APACHE IV
ROD. The mean (± SD) APACHE IV ROD was 0.3 ± 0.3 in both controls
and readmitted group (P=0.84). Despite the APACHE IV score and ROD
being matched, there was a statistically significant difference in
the mean APACHE IV–predicted LOS between the groups (5.4 ± 2.2
days in controls vs 4.9 ± 2.2 days in the readmitted group;
P=0.01).
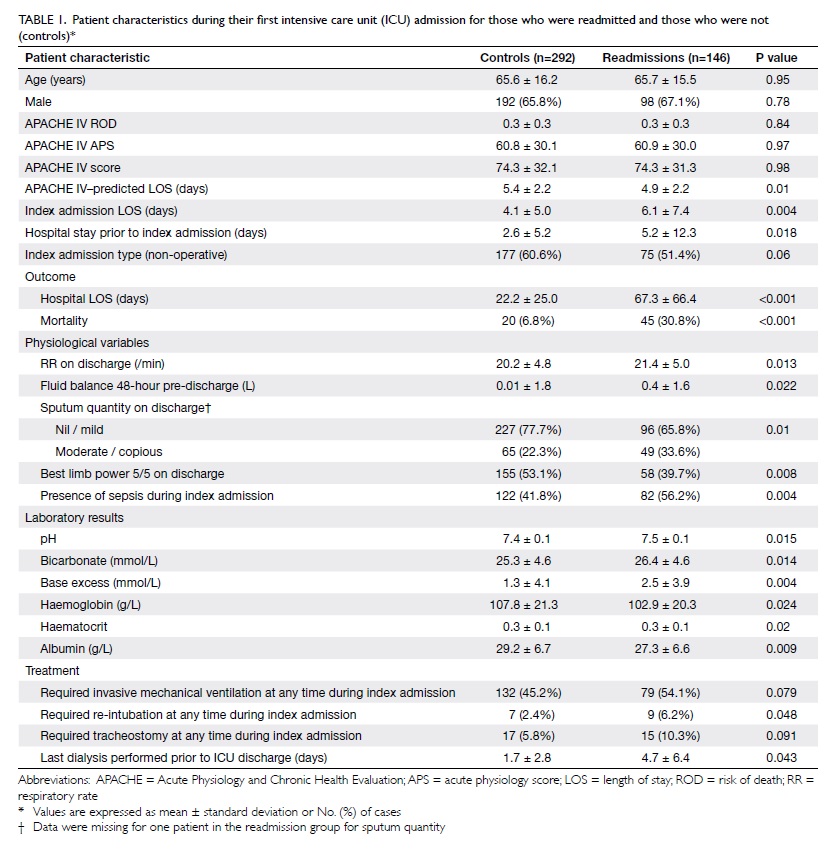
Table 1. Patient characteristics during their first intensive care unit (ICU) admission for those who were readmitted and those who were not (controls)*
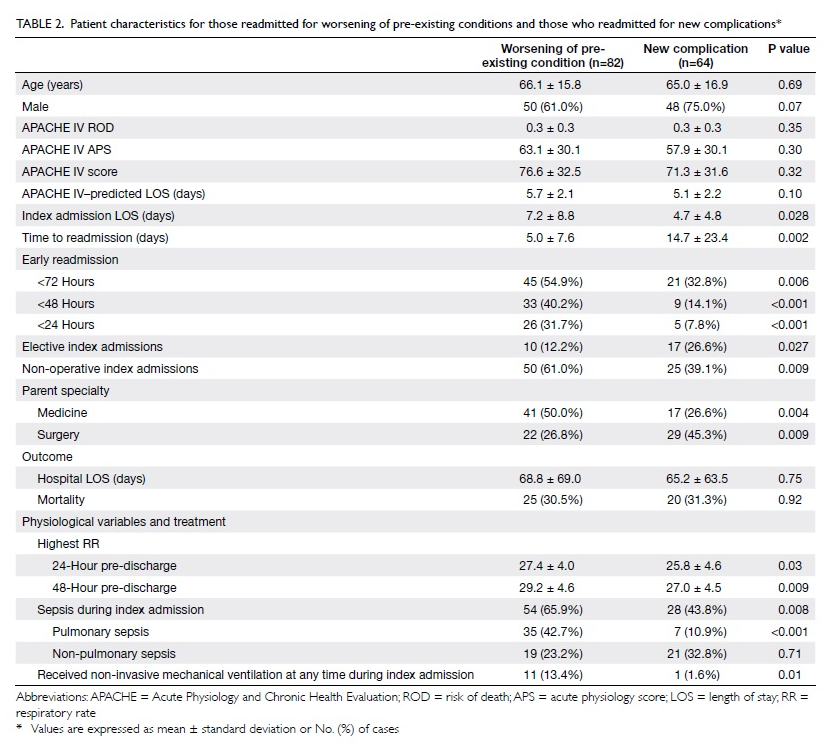
Table 2. Patient characteristics for those readmitted for worsening of pre-existing conditions and those who readmitted for new complications*
Incidents, patient demographics, and
organisational factors
During this 30-month period, 3202 patients
were admitted to the ICU, 380 of whom died in the ICU (361 during
their first ICU admission). Of the 2841 patients discharged from
the ICU alive following their first ICU stay, 146 went on to have
another unplanned ICU admission (ie readmission). Of the 2643
non-readmitted eligible patients who were discharged, 292 were
used as matched controls (Fig 1). Thus the unplanned readmission rate
was 5.1% (146/2841) among patients surviving their first ICU
admission, and the early (within 72 hours) unplanned readmission
rate was 2.3% (66/2841). In our case-control cohort (146
readmissions + 292 controls = 438), 191 (43.6%) patients were from
general wards, 186 (42.5%) were from operating theatres, 52
(11.9%) were direct admissions from the emergency department, and
the remaining admissions were from other sources including
coronary care unit and other hospitals. There were 187 (42.7%)
medical patients, 146 (33.3%) were surgical and 71 (16.2%) were
neurosurgical patients. Of the 438 patients, 363 (82.9%) were
emergency admissions.
Among the 146 readmitted patients, 36
(24.7%) had neurological diseases, 35 (24.0%) had
gastro-intestinal diseases, and 28 (19.2%) had respiratory
diseases as their initial/primary admission diagnosis. Readmitted
patients had spent significantly more days in hospital than
controls prior to their index admissions (5.2 ± 12.3 vs 2.6 ± 5.2
days; P=0.018; Table 1). Self-care ability before ICU
admission and presence of co-morbidities did not differ
significantly in the two groups.
Of the 146 unplanned readmitted patients,
66 (45.2%) were early readmissions (within 72 hours of the index
admission discharge), 42 (28.8%) were within 48 hours, and 31
(21.2%) within 24 hours. The overall readmission rate for daytime
discharges was 5.2% (130/2500), while for nighttime discharges it
was 5.1% (16/314). The early readmission rate for daytime
discharges was 2.3% (57/2500), while for nighttime discharges it
was 2.9% (9/314). The ICU occupancy and nighttime discharges did
not have a significant impact on overall readmissions (P=0.844)
and readmissions within 72 hours (P=0.096). Higher ICU occupancy
was significantly associated with early readmissions (within 48
and 24 hours), compared with late readmissions beyond 48 and 24
hours (t test, P=0.029 and 0.049, respectively).
Reasons for readmission and patient outcomes
Among the unplanned readmissions (n=146),
53 (36.3%) were for respiratory causes, 82 (56.2%) for worsening
of pre-existing conditions, and 64 (43.8%) for new complications.
Among the 82 patients with worsening of pre-existing conditions,
22 (26.8%) had a respiratory admission diagnosis compared to 6/64
(9.4%) who were readmitted for new complications (P=0.008).
Postoperative patients accounted for 32/82 (39.0%) of the patients
readmitted with worsening of pre-existing conditions, as opposed
to 39/64 (60.9%) who were readmitted for new complications
(P=0.009).
Compared with patients readmitted for new
complications, those readmitted for worsening of pre-existing
conditions had significantly longer mean (± SD) index ICU LOS
durations (7.2 ± 8.8 vs 4.7 ± 4.8 days; P=0.028) and shorter mean
times to readmission (5.0 ± 7.6 vs 14.7 ± 23.4 days; P=0.002).
Among those who were readmitted for worsening of pre-existing
conditions, the highest proportion was for respiratory problems
(36/82, 43.9%). The reasons for readmission for new complications were diverse, but respiratory problems were
still the most common (17/64, 26.6%).
Patient outcomes in terms of hospital
mortality and mean hospital LOS were significantly worse in the
readmitted group, despite being matched for initial severity (Table
1). The difference in outcomes in patients readmitted for
worsening of pre-existing conditions or new complications was not
statistically significant (Table 2). Patients readmitted early within
72 hours (13/66, 19.7%) had significantly lower mortality than
those readmitted beyond 72 hours (32/80, 40%; P=0.008).
Risk factors for readmission
Significant findings in the univariate
analysis comparing readmissions and controls are shown in Table
1. Factors examined that were not significant included
admission type (elective or emergency), admission source;
self-care ability before ICU admission; presence of
co-morbidities; admission diagnosis; ICU discharge time; ICU
occupancy on discharge day; mean arterial blood pressure, heart
rate, fractional inspired oxygen (FiO2), Glasgow Coma
Scale (GCS) score on discharge; partial pressure of carbon dioxide
in arterial blood, partial pressure of oxygen in arterial blood
(PaO2), white cell count, platelet count, clotting
profile, and serum levels of urea, creatinine, and total bilirubin
on discharge; whether any anti-arrhythmic agents, inotropic
agents, invasive mechanical ventilation, non-invasive ventilation
(NIV), tracheostomy, dialysis given at any time during index
admission; intubation time; and time from extubation to discharge.
Characteristics of patients readmitted for worsening of
pre-existing problems and for new complications are shown in Table
2. Patients readmitted for worsening of pre-existing
problems had higher mean respiratory rates pre-discharge; more
sepsis (especially pulmonary), and more likely to receive NIV.
Similarly, patients readmitted early (within 72 hours) also had
higher respiratory rates on discharge and were more likely to
receive NIV than those readmitted late.
Factors identified as predisposing to ICU
readmissions in the multivariate logistic regression were:
positive fluid balance in the last 48 hours of the index
admission, higher base excess on discharge, and longer hospital
stays prior to the index admission (Table 3). Other covariates included: index
admission LOS; admission type (postoperative or non-operative);
physiological variables including respiratory rate, cardiac
rhythm, sputum quantity, and best limb power on discharge;
presence of sepsis during the index admission; haematocrit (HCT)
on discharge; treatment including mechanical ventilation,
re-intubation and tracheostomy during the index admission; and
time to last dialysis prior to ICU discharge. Serum albumin values
on discharge were excluded, because missing data exceeded 15%.
Classification tree analysis
Tree model 1 shows the determinant factors
associated with ICU readmission (Fig 2a). The most significant predictor was
whether or not the patient suffered from sepsis during the index
admission (adjusted P=0.004, χ2 = 8.093). Patients with
postoperative sepsis (adjusted P=0.043, χ2 = 4.086),
and non-operative sepsis with fluid gain on discharge (adjusted
P=0.013, χ2 = 13.181) increased the readmission risk
further. For non-septic patients, sputum quantity on discharge had
a significant impact on readmissions (adjusted P=0.006, χ2
= 7.528). Tree model 2 demonstrates that septic patients without
full limb power at discharge from the ICU had a higher risk of
deterioration than those with any other pre-existing condition (Fig 2b). In contrast to readmissions due to
new complications, postoperative patients with a HCT of ≤0.34 were
at highest risk (Tree model 3, Fig 2c).
Discussion
In our cohort, 5.1% of those who survived
their first ICU admission were readmitted to the ICU; early
readmissions amounted to 2.3%. The outcome of readmitted patients
was significantly worse than that of those not readmitted, despite
being matched for illness severity in terms of APACHE ROD when
initially admitted to the ICU. This outcome discrepancy signifies
the importance of identifying patients at high risk of
deterioration after initial discharge from intensive care. The
readmitted group had a significantly shorter APACHE IV–predicted
LOS than the controls. Despite this, the actual ICU LOS in the
controls was shorter than predicted, while in the readmitted
group, it was longer than predicted. This suggested that despite
being matched for initial severity, readmitted patients had poorer
responses to treatment or had already endured longer initial ICU
stays. Not surprisingly, delay in ICU admission increased a
patient's risk of readmission; readmitted patients had
significantly longer mean values for hospital LOS prior to their
index ICU admission, apart from being a significant predictor of
ICU readmission in the multivariate analysis. Our study also
demonstrated that patients readmitted for worsening of
pre-existing conditions and for new complications had different
characteristics, but comparable outcomes.
The influence of pulmonary status on the
risk of readmission is not debated. Previous studies found
pulmonary disorder to be the leading cause of readmissions.1 3 7 15 20 21 The effect of sputum quantity on readmission
was likely attributable to insufficient cough effort and retention
of secretions by patients. Critically ill patients with
neuromuscular complications from severe polyneuropathy and
myopathy or deconditioning and weakness were at great risk of
sputum retention and nosocomial pneumonia. They were also at risk
of hypoventilation and type 2 respiratory failures.22 23
Similar findings were reported in patients with severe head
trauma.24 25 In our cohort, patients with neurological
diseases constituted the highest proportion of readmissions.
Resource allocation for early rehabilitation in the ICU might be
warranted.23 Good airway
and pulmonary care is crucial for post-discharge patients in
step-down units. On the other hand, reducing ventilator-associated
pneumonia (VAP) rates by adhering to VAP prevention bundles during
the ICU stays may be a way to reduce readmission rates.26 27
Another finding in this study was the
effect of fluid balance in the pre-discharge period. Previous
studies have illustrated the association of fluid overloading and
deleterious outcomes in critically ill patients, including those
with sepsis,28 acute
kidney injury,29 acute
lung injury,28 30 and following operations.31 A single-centre study in Japan32 found that
weight gain at the time of initial ICU discharge had a negative
linear relationship with the time to ICU readmission, as well as
PaO2-to-FiO2 ratio.
As vigorous fluid resuscitation is often necessary in the initial
management of patients with critical illnesses, a proportion of
those readmitted to the ICU with respiratory failure could have
experienced lung oedema or atelectasis. The current study supports
the finding that discharging patients with positive fluid balance
leads to a higher readmission rate. Diuresis in critically ill
patients could be recognised as a sign of recovery from their
illness.
The association of HCT values at discharge
and readmission was reported in previous studies, but a cutoff
predictive value had not been specified.4
7 In the tree analysis of
the subgroup readmitted for new complications, postoperative
patients with HCTs of ≤0.34 were associated with an increased risk
of readmission. The corresponding haemoglobin levels in patients
with HCTs of 0.34 ranged between 110 and 120 g/L. Many confounders
complicate the interpretation of HCT. In our cohort, control and
readmitted patients were matched for age, gender, and initial
disease severity. Thus, lower HCTs in the readmitted group could
represent a more severe illness upon ICU discharge or more
haemodilution. Yet, according to current transfusion practice in
critically ill patients (based on the Transfusion Requirements in
Critical Care study), outcomes in those with a restrictive
transfusion threshold (7 g/L) were at least equivalent to using a
liberal threshold (10 g/L).33
In critically ill patients, observational studies have shown a
significant association of red cell transfusions with mortality.34 However, in a more
recent multicentred study in Europe,35 an extended Cox proportional
hazards analysis showed that patients who received transfusion in
fact enjoyed better survival.
These contradictory findings remind us that there is no single
value for the haemoglobin concentration that justifies
transfusion. Patients with poor cardiopulmonary reserve might
benefit from a more liberal transfusion threshold.34 In our cohort, postoperative patients with
lower HCT values were most vulnerable to new complications that
warranted ICU readmission. The stress of major operations to the
cardiopulmonary status of an anaemic patient should not be
overlooked.
The influence of base excess on readmission
was observed in the logistic regression model. Common causes of
alkalosis in critically ill patients include contraction alkalosis
and renal compensation for respiratory acidosis. It is
hypothesised that the majority of our patients with alkalosis were
post-hypercapnic and higher readmission rates were seen in
patients with more severe hypercapnia on initial presentation. On
the other hand, 45% of patients in our cohort were discharged with
alkalosis (arterial pH >7.45), whilst only 3.4% (n=15) were
discharged with acidosis (arterial pH <7.35). This reflects the
tendency to avoid discharging patients with acidosis in our daily
practice.
A few previous studies identified the GCS
score upon discharge as a risk factor for ICU readmission.5 18 On
the contrary, we found that whether or not a patient was
discharged with full limb power predicted readmission for
worsening pre-existing conditions. We hypothesise that a patient's
GCS score upon ICU discharge reflects initial ICU admission
severity and status, which was actually matched in our study. For
example, a patient admitted with a low GCS score (and thus higher
disease severity) is more likely to be discharged with a lower GCS
score.
Strengths and limitations
Our case-control design enabled extensive
data collection on pre-discharge status. Many of the collected
variables have not been reported on previously. In the current
study, readmitted and non-readmitted patients were matched for
initial severity of illness in terms of APACHE IV ROD. Data
collection was focused on the variables that occurred after ICU
admission and were modifiable. However, variables reflecting
initial disease severity and associated with readmission might
have been overlooked. Moreover, the data abstraction and
categorisation processes were not blinded to the outcome status of
the subjects, and were therefore prone to information bias. Our
study did not take into account the proportion of patients who had
a poor physician-predicted chance of long-term survival and were
therefore not readmitted. As this was a single-centre cohort, the
importance of differences in case-mix and patterns of readmission
in different ICUs should be recognised.
To the best of our knowledge, this was the
first study employing the classification tree for analysis of ICU
readmissions. Logistic regression is valuable in providing an
indication of the relative importance of each predictor.
Higher-order interactions between the predictor variables could be
demonstrated in the classification tree analysis. If interactions
between independent variables were present, the results of the
multiple logistic regression might not be valid. By contrast,
factors identified using the tree models might only have an
important influence in specific subgroups. For example, the
association of sputum quantity with readmission could be hidden if
we considered all patients, but not among non-septic patients
(Tree model 1).
Conclusion
Our cohort was consistent with previous
studies, and suggested that patients having ICU readmissions had
significantly poorer outcomes in terms of hospital mortality and
hospital LOS. The characteristics of patients readmitted for
worsening of pre-existing conditions and for new complications
appeared to differ. Incomplete resolution of respiratory
conditions remained an important reason for potentially
preventable ICU readmission. Attention to patients' fluid balance
and sputum quantity before ICU discharge might help to prevent
unplanned ICU readmissions. Further study is warranted to
investigate the effect of the HCT and pH on critically ill
patients.
References
1. Rosenberg AL, Hofer TP, Hayward
RA, Strachan C, Watts CM. Who bounces back? Physiologic and other
predictors of intensive care unit readmission. Crit Care Med
2001;29:511-8. CrossRef
2. Rosenberg AL, Watts C. Patients
readmitted to ICUs*: a systematic review of risk factors and
outcomes. Chest 2000;118:492-502. CrossRef
3. Franklin C, Jackson D. Discharge
decision-making in a medical ICU: characteristics of unexpected
readmissions. Crit Care Med 1983;11:61-6. CrossRef
4. Rubins HB, Moskowitz MA.
Discharge decision-making in a medical intensive care unit.
Identifying patients at high risk of unexpected death or unit
readmission. Am J Med 1988;84:863-9. CrossRef
5. Kramer AA, Higgins TL, Zimmerman
JE. Intensive care unit readmissions in U.S. hospitals: patient
characteristics, risk factors, and outcomes. Crit Care Med
2012;40:3-10. CrossRef
6. Yoon KB, Koh SO, Han DW, Kang
OC. Discharge decision-making by intensivists on readmission to
the intensive care unit. Yonsei Med J 2004;45:193-8.
7. Durbin CG Jr, Kopel RF. A
case-control study of patients readmitted to the intensive care
unit. Crit Care Med 1993;21:1547-53. CrossRef
8. Alban RF, Nisim AA, Ho J, Nishi
GK, Shabot MM. Readmission to surgical intensive care increases
severity-adjusted patient mortality. J Trauma 2006;60:1027-31. CrossRef
9. Chan KS, Tan CK, Fang CS, et al.
Readmission to the intensive care unit: an indicator that reflects
the potential risks of morbidity and mortality of surgical
patients in the intensive care unit. Surg Today 2009;39:295-9. CrossRef
10. Priestap FA, Martin CM. Impact
of intensive care unit discharge time on patient outcome. Crit
Care Med 2006;34:2946-51.
11. Baigelman W, Katz R, Geary G.
Patient readmission to critical care units during the same
hospitalization at a community teaching hospital. Intensive Care
Med 1983;9:253-6. CrossRef
12. Cooper GS, Sirio CA, Rotondi
AJ, Shepardson LB, Rosenthal GE. Are readmissions to the intensive
care unit a useful measure of hospital performance? Med Care
1999;37:399-408. CrossRef
13. Berenholtz SM, Dorman T, Ngo
K, Pronovost PJ. Qualitative review of intensive care unit quality
indicators. J Crit Care 2002;17:1-12. CrossRef
14. Metnitz PG, Fieux F, Jordan B,
Lang T, Moreno R, Le Gall JR. Critically ill patients readmitted
to intensive care units—lessons to learn? Intensive Care Med
2003;29:241-8.
15. Campbell AJ, Cook JA, Adey G,
Cuthbertson BH. Predicting death and readmission after intensive
care discharge. Br J Anaesth 2008;100:656-62. CrossRef
16. Ho KM, Dobb GJ, Lee KY, Finn
J, Knuiman M, Webb SA. The effect of comorbidities on risk of
intensive care readmission during the same hospitalization: a
linked data cohort study. J Crit Care 2009;24:101-7. CrossRef
17. Frost SA, Alexandrou E,
Bogdanovski T, et al. Severity of illness and risk of readmission
to intensive care: a meta-analysis. Resuscitation 2009;80:505-10. CrossRef
18. Gajic O, Malinchoc M, Comfere
TB, et al. The stability and workload index for transfer score
predicts unplanned intensive care unit patient readmission:
initial development and validation. Crit Care Med 2008;36:676-82. CrossRef
19. Yates JW, Chalmer B, McKegney
FP. Evaluation of patients with advanced cancer using the
Karnofsky performance status. Cancer 1980;45:2220-4. CrossRef
20. Chen LM, Martin CM, Keenan SP,
Sibbald WJ. Patients readmitted to the intensive care unit during
the same hospitalization: clinical features and outcomes. Crit
Care Med 1998;26:1834-41. CrossRef
21. Snow N, Bergin KT, Horrigan
TP. Readmission of patients to the surgical intensive care unit:
patient profiles and possibilities for prevention. Crit Care Med
1985;13:961-4. CrossRef
22. Hermans G, De Jonghe B,
Bruyninckx F, Van den Berghe G. Interventions for preventing
critical illness polyneuropathy and critical illness myopathy.
Cochrane Database Syst Rev 2009;(1):CD006832.
23. Latronico N, Bolton CF.
Critical illness polyneuropathy and myopathy: a major cause of
muscle weakness and paralysis. Lancet Neurol 2011;10:931-41. CrossRef
24. Sirgo G, Bodi M, Díaz E, Rello
J. Pneumonia in head-injured and severe trauma patients. Semin
Respir Crit Care Med 2002;23:435-41. CrossRef
25. Cavalcanti M, Ferrer M, Ferrer
R, Morforte R, Garnacho A, Torres A. Risk and prognostic factors
of ventilator-associated pneumonia in trauma patients. Crit Care
Med 2006;34:1067-72. CrossRef
26. Morris AC, Hay AW, Swann DG,
et al. Reducing ventilator-associated pneumonia in intensive care:
impact of implementing a care bundle. Crit Care Med
2011;39:2218-24. CrossRef
27. O'Keefe-McCarthy S, Santiago
C, Lau G. Ventilator-associated pneumonia bundled strategies: an
evidence-based practice. Worldviews Evid Based Nurs
2008;5:193-204. CrossRef
28. Wiedemann HP, Wheeler AP,
Bernard GR, et al. Comparison of two fluid-management strategies
in acute lung injury. N Engl J Med 2006;354:2564-75. CrossRef
29. Bouchard J, Mehta RL. Fluid
accumulation and acute kidney injury: consequence or cause. Curr
Opin Crit Care 2009;15:509-13. CrossRef
30. Sakr Y, Vincent JL, Reinhart
K, et al. High tidal volume and positive fluid balance are
associated with worse outcome in acute lung injury. Chest
2005;128:3098-108. CrossRef
31. Stewart RM, Park PK, Hunt JP,
et al. Less is more: improved outcomes in surgical patients with
conservative fluid administration and central venous catheter
monitoring. J Am Coll Surg 2009;208:725-35; discussion 735-7. CrossRef
32. Matsuoka Y, Zaitsu A,
Hashizume M. Investigation of the cause of readmission to the
intensive care unit for patients with lung edema or atelectasis.
Yonsei Med J 2008;49:422-8. CrossRef
33. Hébert PC, Wells G, Blajchman
MA, et al. A multicenter, randomized, controlled clinical trial of
transfusion requirements in critical care. Transfusion
Requirements in Critical Care Investigators, Canadian Critical
Care Trials Group. N Engl J Med 1999;340:409-17. CrossRef
34. Marik PE, Corwin HL. Efficacy
of red blood cell transfusion in the critically ill: a systematic
review of the literature. Crit Care Med 2008;36:2667-74. CrossRef
35. Vincent JL, Sakr Y, Sprung C,
Harboe S, Damas P. Are blood transfusions associated with greater
mortality rates? Results of the sepsis occurrence in acutely ill
patients study. Anesthesiology 2008;108:31-9. CrossRef