Hong Kong Med J 2023 Dec;29(6):557–60 | Epub 11 Dec 2023
© Hong Kong Academy of Medicine. CC BY-NC-ND 4.0
COMMENTARY
Large language models: implications of rapid evolution in medicine
Billy HH Cheung, MEd, FCSHK (Gen); Michael TH Co, MS, FCSHK (Gen)
Department of Surgery, School of Clinical Medicine, Li Ka Shing Faculty of Medicine, The University of Hong Kong, Hong Kong SAR, China
Corresponding author: Dr Michael TH Co (mcth@hku.hk)

Introduction
Large language models (LLMs), a kind of generative
artificial intelligence, have ignited considerable
interest since the release of OpenAI’s GPT-3.5
model in November 2022.1 These complex models
have incited substantive dialogue within scientific
communities due to their potential applications in
various fields, including medicine. For example, a
previous investigation confirmed ChatGPT’s ability
to pass medical, legal, and business examinations
with ease.2 3 Nonetheless, in a study by Salvagno et al,4
its limitations were revealed due to two major
issues. First, a phenomenon referred to as ‘artificial
hallucination’ arises where the LLM generates
information that appears realistic but is, in fact,
entirely fabricated and without any factual foundation
due to imperfect training data and algorithms.4
Second, in the case of ChatGPT, its dataset being
limited to information up to September 2021.5
However, breakthroughs are being made as
these LLMs are updated to overcome these shortfalls.
Shortly after the publication by Salvagno et al4
in which the GPT-3.5 model was used, the GPT-4
model was introduced on 14 March 2023, and
demonstrated improved accuracy due to a larger
dataset and multimodal functionality.6 This was
further improved with a web-browsing function that
was introduced on 13 May 2023, enabling almost
borderless information access.7 These two major
upgrades provided new abilities to ChatGPT that go
some way towards overcoming its prior limitations.
Hence, we have re-evaluated the performance
and reliability of ChatGPT and compared these
results with the findings of the original study by
Salvagno et al.4
Results with the GPT-4 model with web access versus the GPT-3.5 model
By employing the same prompt used in the study
by Salvagno et al (online supplementary Appendix
1),4 we observed that the GPT-4 model was able to
locate, analyse, and summarise the articles8 9 10 in just
3 minutes and 42 seconds over a standard domestic internet connection. The model demonstrated its
capability to accurately summarise studies and
engage in an ongoing dialogue to examine the
topic comprehensively (online supplementary
Appendix 2). Remarkably, the model could also
generate code for Visual Basic for Applications
in Microsoft PowerPoint, thereby facilitating
the near-autonomous creation of a slide with a
summary table (Fig8 9 10). On reviewing the original
research articles, we affirmed the accuracy of
ChatGPT’s outputs. Compared with the response
generated by the GPT-3.5 model in the article by
Salvagno et al,4 which was completely fabricated,
the improvements are notable. The output of Visual
Basic for Applications also demonstrates the ability
of ChatGPT to write programming code, which helps
automate presentation preparation and improve the
efficiency of information distribution. This confirms
our hypothesis that the GPT-4 model contains
significant enhancements that bring numerous
potential applications in the field of medicine.
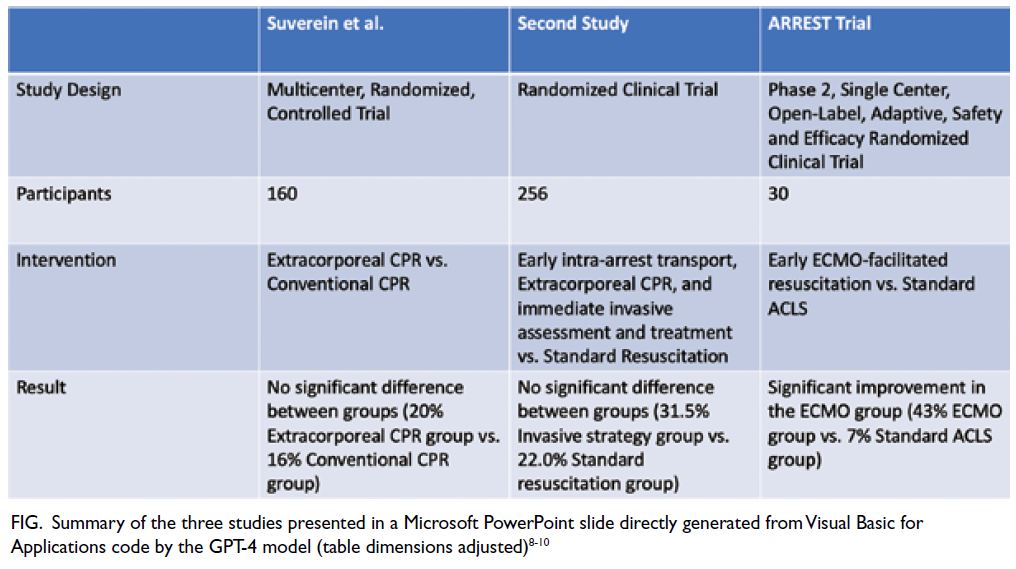
Figure. Summary of the three studies presented in a Microsoft PowerPoint slide directly generated from Visual Basic for Applications code by the GPT-4 model (table dimensions adjusted)8 9 10
Recent evolution of large language
models
The trajectory of LLM development in recent years
has been marked by significant advancements in
functionality and sophistication, as covered in other
major articles.11 12 The investigation by Salvagno et al,4
which used the GPT-3.5 model, revealed several
limitations, raising questions about the applicability
and reliability of LLMs in critical fields such as clinical
medicine and scientific research. Nonetheless, the
introduction of the GPT-4 model represented a
key milestone. This new iteration brought a suite of
enhancements, including improved accuracy and
multimodal functionality, broadening the range of
data types the model could interrogate, process,
and integrate2 and thereby expanding the potential
applications of LLMs.
In a further leap, the developers incorporated
a web-browsing function that allows the model to
access information beyond its built-in knowledge
base, making it a more robust and versatile
tool. In addition, many developers have begun
building plugins to ChatGPT to allow access to other information sources, such as PDF (Portable Document Format) documents and specific databases.13
These advancements necessitate detailed
and repeated assessment of the performance and
reliability of LLMs. As the models continue to evolve,
this continuous process of review and refinement is
crucial to maximising their potential applications
in medicine while also addressing their inherent
limitations.
Utilisation of large language models in medicine
The potential applications of LLMs in medicine
are numerous and may bring forth transformative
changes not only in clinical research but also in
practical settings and medical education. For
example, because a substantial portion of clinical
information is text-based, LLMs could easily be
used to enhance the efficacy and precision of patient
summaries and referrals.14 15 Moreover, by processing
extensive text-based medical knowledge, LLMs could
serve as invaluable clinical assistants. Recent local
research has corroborated this by demonstrating
how such models can improve diagnostic confidence
and early commencement of appropriate treatment
in medicine, even in complicated geriatric patients.16
The use of LLMs should improve work efficiency
and accuracy, which in turn would allow clinicians
to spend more time with patients, thereby improving
communication, trust, and the standard of care.
Medical education represents another domain where the use of LLMs holds promise. The escalating
demand for superior medical education stems from
the growing need for more proficient doctors and
the continuous expansion of medical knowledge
across various specialties. Large language models
can contribute to this by acting as teaching assistants
for educators and providing customised learning
support for students.17 For example, our recent
work demonstrated the effectiveness of LLMs in the
creation of examination questions.18 This suggests
that LLMs can also play a pivotal role as learning
aids for medical students, enabling tailored learning
experiences and promoting active recall, thereby
enriching the educational journeys of future doctors.
Limitations of the current GPT-4 model
While the advancements are promising and rapid,
the current state of LLMs is still far from perfect.
It took our team five attempts to obtain a summary
of all three articles; in the other four attempts,
ChatGPT failed to access the link for one of the
articles, and on one occasion, it attempted to search
for similar articles to finish its task. On examining
the conversation and final output, some may argue
that the output from ChatGPT lacks depth and
insight. However, we did not encounter any profound
hallucinations during the process, and the limitations
we observed can be attributed to restricted access to
the original articles and the fact that ChatGPT has to
look for information from other sources.
In summary, the limitations of the GPT-4 model are still apparent. Nonetheless, the latest version might approach the capabilities of a junior resident or research assistant when performing
similar tasks. The limitations might be overcome
by allowing access to full articles, increasing the
word limit of queries, and allowing more time for
additional training, which all seem possible.
Future possibilities
The speed and accuracy of knowledge synthesis that
LLMs offer has transformative potential for research,
clinical care, and education. Perhaps in a few years,
a doctor not utilising LLMs will be seen as outdated,
akin to how someone who does not use the internet
might be viewed now. Moor et al19 have proposed
that, with the availability of LLMs and multimodal
models, foundation generalist medical artificial
intelligence models, developed from general LLMs
with access to clinical training datasets, may be on
the horizon. While many can see the potential of
LLMs, others fear that their rapid development could
jeopardise our role as physicians. A few years ago,
AlphaGo, an artificial intelligence system that plays
the board game Go and was trained by humans with
supervised and reinforcement learning, defeated the
world’s strongest human players 60 games to zero
in January 2017.20 Not long after, AlphaGo Zero, a
similar system that taught itself to play Go without
human supervision, triumphed over the earlier
version. Researchers found that the programme
developed tactics that no human had discovered
before. In a similar manner, could current LLMs,
built upon artificial intelligence programmes with
machine learning, follow a similar trajectory and
surpass humans in the near future?
Potential for inequity and discrimination
Looking beyond the promising possibilities of
LLMs, we can also see the potential for engendering
inequality through uneven access. For instance, in
regions like Hong Kong, where access restrictions on
such models have been imposed,21 users must resort
to virtual private networks to access these resources.
Financial constraints further exacerbate
this inequality. More advanced iterations, such
as the GPT-4 model, are exclusively available to
paid subscribers. This creates a financial barrier
by denying access to those unable to afford the
subscription fees.
In addition, there is the contentious issue of
preferential access, wherein certain institutions are
granted early access to novel features before public
release. This effectively tilts the playing field, creating
an undue advantage for a select few.
When LLMs such as ChatGPT evolve into
effective and potent tools, these disparities may
incite social injustice. Moreover, such imbalances could further widen the scientific, technological,
and economic progress gaps between different
stakeholders, underscoring the urgent need for
equitable distribution and access to these tools.
Conclusion
We are at a pivotal juncture in history where artificial
intelligence and LLMs are becoming increasingly
reliable and usable tools with the potential to
revolutionise clinical practice, scientific discovery,
and teaching. We encourage clinicians and
researchers to harness the power of these tools, think
creatively about their applications, and continually
explore ways in which they can enhance our work,
drive scientific advancements, and ultimately benefit
humanity as a whole.
Author contributions
Concept or design: BHH Cheung.
Acquisition of data: Both authors.
Analysis or interpretation of data: Both authors.
Drafting of the manuscript: BHH Cheung.
Critical revision of the manuscript for important intellectual content: MTH Co.
Acquisition of data: Both authors.
Analysis or interpretation of data: Both authors.
Drafting of the manuscript: BHH Cheung.
Critical revision of the manuscript for important intellectual content: MTH Co.
Both authors had full access to the data, contributed to the study, approved the final version for publication, and take responsibility for its accuracy and integrity.
Conflicts of interest
Both authors have disclosed no conflicts of interest.
Funding/support
This commentary received no specific grant from any funding agency in the public, commercial, or not-for-profit sectors.
References
1. OpenAI. Introducing ChatGPT. Available from: https://openai.com/blog/chatgpt. Accessed 13 Feb 2023.
2. OpenAI. GPT-4 technical report. Available from: https://arxiv.org/abs/2303.08774. Accessed 20 Nov 2023.
3. Kung TH, Cheatham M, Medenilla A, et al. Performance of ChatGPT on USMLE: potential for AI-assisted medical education using large language models. PLOS Digit Health 2023;2:e0000198. Crossref
4. Salvagno M, Taccone FS, Gerli AG. Artificial intelligence hallucinations. Crit Care 2023;27:180. Crossref
5. Alkaissi H, McFarlane SI. Artificial hallucinations in ChatGPT: implications in scientific writing. Cureus 2023;15:e35179. Crossref
6. OpenAI. GPT-4. Available from: https://openai.com/research/gpt-4. Accessed 16 Mar 2023.
7. Mok A. OpenAI is rolling out a game-changing feature to
ChatGPT this week that could revolutionize how we use
the internet. Business Insider. Available from: https://www.businessinsider.com/chatgpt-openai-web-browsing-plug-change-how-we-use-internet-2023-5. Accessed 21 May 2023.
8. Suverein MM, Delnoij TS, Lorusso R, et al. Early extracorporeal CPR for refractory out-of-hospital cardiac arrest. New Engl J Med 2023;388:299-309. Crossref
9. Belohlavek J, Smalcova J, Rob D, et al. Effect of intra-arrest
transport, extracorporeal cardiopulmonary resuscitation,
and immediate invasive assessment and treatment on
functional neurologic outcome in refractory out-of-hospital
cardiac arrest. JAMA 2022;327:737-47. Crossref
10. Yannopoulos D, Bartos J, Raveendran G, et al. Advanced
reperfusion strategies for patients with out-of-hospital
cardiac arrest and refractory ventricular fibrillation
(ARREST): a phase 2, single centre, open-label, randomised
controlled trial. Lancet 2020;396:1807-16. Crossref
11. Sallam M. ChatGPT utility in healthcare education,
research, and practice: systematic review on the promising
perspectives and valid concerns. Healthcare (Basel)
2023;11:887. Crossref
12. Cascella M, Montomoli J, Bellini V, Bignami E. Evaluating
the feasibility of ChatGPT in healthcare: an analysis
of multiple clinical and research scenarios. J Med Syst
2023;47:33. Crossref
13. OpenAI. ChatGPT plugins. Available from: https://doi.org/10.3390/healthcare11060887. Accessed 4 Jun 2023.
14. Ali SR, Dobbs TD, Hutchings HA, Whitaker IS. Using
ChatGPT to write patient clinic letters. Lancet Digit Health 2023;5:e179-81. Crossref
15. Patel SB, Lam K. ChatGPT: the future of discharge
summaries? Lancet Digit Health 2023;5:e107-8. Crossref
16. Shea YF, Lee CM, Ip WC, Luk DW, Wong SS. Use of GPT-4
to analyze medical records of patients with extensive
investigations and delayed diagnosis. JAMA Netw Open
2023;6:e2325000. Crossref
17. Grigorian A, Shipley J, Nahmias J, et al. Implications of
using chatbots for future surgical education. JAMA Surg
2023;158:1220-2. Crossref
18. Cheung BH, Lau GK, Wong GT, et al. ChatGPT versus
human in generating medical graduate exam multiple
choice questions—a multinational prospective study
(Hong Kong S.A.R., Singapore, Ireland, and the United
Kingdom). PLoS One 2023;18:e0290691. Crossref
19. Moor M, Banerjee O, Abad ZS, et al. Foundation models
for generalist medical artificial intelligence. Nature
2023;616:259-65. Crossref
20. Silver D, Schrittwieser J, Simonyan K, et al. Mastering
the game of Go without human knowledge. Nature
2017;550:354-9. Crossref
21. OpenAI. Supported countries and territories. Available
from: https://platform.openai.com/docs/supported-countries. Accessed 22 May 2023.